How Can Big Data Help Detect and Prevent Fraud
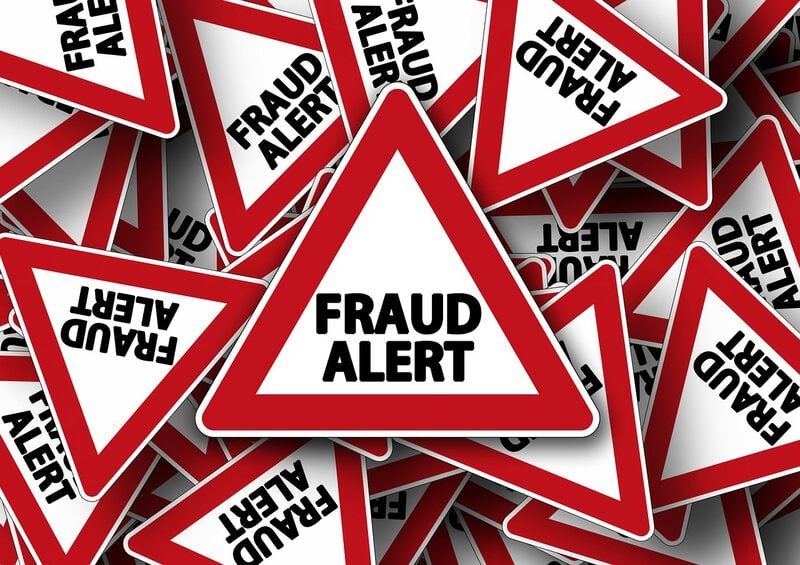
This article examines the potential of big data in the detection and prevention of fraud.
It explores the role of big data in uncovering fraudulent activities and highlights techniques for analyzing large datasets to identify fraudulent patterns.
Additionally, the article discusses the use of machine learning algorithms and advanced analytics tools for fraud detection and prevention.
Furthermore, it delves into the importance of real-time monitoring and alert systems in mitigating fraud risks.
Key Takeaways
- Big data analytics revolutionizes fraud prevention techniques by analyzing large volumes of data in real-time.
- Techniques such as anomaly detection, network analysis, and predictive modeling help identify patterns and anomalies indicating fraudulent behavior.
- Machine learning algorithms enhance organizations’ capabilities in detecting and preventing fraud by analyzing historical data and adapting to new data.
- Advanced analytics tools and technologies enable real-time monitoring and analysis, allowing organizations to take proactive measures to prevent fraud.
The Role of Big Data in Fraud Detection and Prevention
The role of big data in fraud detection and prevention is a topic of interest in the field of data analytics. Big data applications have revolutionized the way fraud prevention techniques are implemented. With the increasing amount of data generated by businesses and individuals, traditional methods of fraud detection have become inadequate.
Big data analytics provide the capability to analyze large volumes of data in real-time, enabling organizations to identify patterns and anomalies associated with fraudulent activities. By incorporating advanced algorithms and machine learning techniques, big data can help identify potential fraud cases more efficiently and accurately.
Furthermore, big data can also be used to develop predictive models that can anticipate and prevent fraudulent activities before they occur. This proactive approach to fraud prevention has the potential to save businesses significant financial losses and protect the interests of customers.
Techniques for Analyzing Big Data to Uncover Fraudulent Activities
One approach to uncovering fraudulent activities involves employing advanced analytical techniques on large datasets. Fraud detection techniques and data analysis methods are crucial in identifying patterns and anomalies that may indicate fraudulent behavior.
These techniques involve the use of statistical models, machine learning algorithms, and data visualization tools to analyze vast amounts of data and identify suspicious activities. Common fraud detection techniques include anomaly detection, network analysis, and predictive modeling.
Anomaly detection involves identifying unusual patterns or outliers in the data that may indicate fraudulent behavior. Network analysis focuses on identifying connections and relationships among entities to detect potential fraud networks. Predictive modeling uses historical data to build models that can predict future fraudulent activities.
Leveraging Machine Learning Algorithms for Fraud Detection
Employing machine learning algorithms in fraud detection aids in the identification of patterns, anomalies, and connections among entities, thereby enhancing organizations’ capabilities in detecting and preventing fraudulent activities. Machine learning techniques have been widely adopted in the field of fraud prevention due to their ability to analyze large volumes of data and uncover hidden patterns that may indicate fraudulent behavior. These techniques leverage algorithms that are trained on historical data to learn the characteristics of fraudulent transactions and distinguish them from legitimate ones. By continuously analyzing new data, machine learning algorithms can adapt and improve their detection capabilities over time. This approach enables organizations to stay ahead of fraudsters and implement more effective fraud prevention strategies.
Below is a table illustrating some common machine learning techniques used in fraud detection:
Technique | Description |
---|---|
Supervised Learning | Algorithms learn from labeled examples to predict if a transaction is fraudulent or legitimate. |
Unsupervised Learning | Algorithms identify patterns and anomalies in the data without prior knowledge of fraud cases. |
Neural Networks | Deep learning models that can capture complex relationships and patterns in the data. |
Ensemble Methods | Combining multiple algorithms to improve accuracy and robustness in fraud detection. |
Big Data Analytics Tools and Technologies for Fraud Prevention
Utilizing advanced analytics tools and technologies enables organizations to leverage large volumes of information and extract valuable insights for enhancing their fraud prevention strategies. Big data analytics techniques play a crucial role in identifying patterns, anomalies, and trends that can indicate potential fraudulent activities. By analyzing vast amounts of structured and unstructured data, organizations can detect suspicious behaviors, identify emerging fraud patterns, and predict future fraudulent activities. This enables them to take proactive measures to prevent fraud before it occurs.
Furthermore, big data analytics tools provide real-time monitoring and analysis capabilities, allowing organizations to continuously monitor transactions, detect suspicious activities in real-time, and respond promptly to mitigate potential risks. By incorporating big data analytics techniques into their fraud prevention strategies, organizations can enhance their ability to detect and prevent fraud effectively.
In summary:
- Big data analytics enables the analysis of large volumes of data to identify patterns and trends related to fraudulent activities.
- Advanced analytics tools can detect suspicious behaviors and emerging fraud patterns.
- Real-time monitoring and analysis capabilities help organizations detect and respond promptly to potential fraud risks.
- Incorporating big data analytics techniques enhances organizations’ ability to detect and prevent fraud effectively.
Real-time Monitoring and Alert Systems for Fraud Prevention
This discussion aims to explore the importance of data-driven fraud detection, the benefits of instant fraud alerts, and the effectiveness of real-time fraud prevention.
In the context of fraud prevention, data-driven techniques play a crucial role in identifying patterns and anomalies that may indicate fraudulent activities.
Instant fraud alerts allow organizations to promptly respond to potential fraud incidents, mitigating the risk and minimizing the potential impact.
Real-time fraud prevention systems leverage advanced technologies and algorithms to detect and prevent fraudulent transactions as they occur, providing an additional layer of security for businesses and individuals.
Data-Driven Fraud Detection
Data-driven fraud detection techniques involve analyzing large datasets to identify patterns and anomalies that can be indicative of fraudulent activities. These techniques have proven to be effective in detecting and preventing fraud in various industries.
Here are four key aspects of data-driven fraud detection:
- Advanced analytics: Data-driven fraud prevention relies on sophisticated analytics algorithms that can process large volumes of data quickly and accurately. These algorithms can detect unusual patterns, outliers, and anomalies that may indicate fraudulent activities.
- Machine learning: Machine learning algorithms can be trained to identify patterns of fraudulent behavior based on historical data. These algorithms can continuously learn and adapt to new fraud patterns, improving the accuracy of fraud detection over time.
- Real-time monitoring: Data-driven fraud detection systems enable real-time monitoring of transactions and activities, allowing for immediate detection and response to potential fraud incidents. This real-time monitoring helps prevent financial losses and mitigate the impact of fraud.
- Integration of diverse data sources: Data-driven fraud detection techniques integrate data from multiple sources, such as transaction records, customer profiles, and external data feeds. By analyzing a wide range of data, these techniques can uncover hidden connections and patterns, enhancing the accuracy of fraud detection.
Overall, data-driven fraud detection techniques play a crucial role in identifying and preventing fraudulent activities, providing organizations with effective tools to safeguard their operations and assets.
Instant Fraud Alerts
Data-driven fraud detection techniques have proved effective in identifying fraudulent activities by analyzing large sets of data. However, relying solely on retrospectively analyzing transactions may not be sufficient to prevent fraud in real-time.
To address this limitation, instant fraud detection systems have been developed. These systems utilize advanced algorithms and machine learning techniques to continuously monitor and analyze incoming transactions in real-time. By instantly flagging suspicious activities, these systems enable organizations to take immediate action to prevent fraudulent transactions from being processed.
To enhance the effectiveness of instant fraud detection, various fraud prevention techniques have been integrated into these systems. These techniques include identity verification, behavior analysis, anomaly detection, and predictive modeling. By combining these techniques with real-time monitoring, instant fraud detection systems provide organizations with a proactive approach to combat fraud and protect their assets.
Real-Time Fraud Prevention
Real-time fraud prevention strategies employ advanced algorithms and machine learning techniques to continuously monitor and analyze incoming transactions, allowing organizations to promptly identify and address suspicious activities. These strategies are characterized by their ability to detect and prevent fraud in real-time, minimizing the potential damage caused by fraudulent transactions.
The use of dynamic risk assessment plays a crucial role in this process, as it enables organizations to evaluate the risk associated with each transaction in real-time, based on various factors such as transaction history, customer behavior patterns, and geographical location.
Big Data’s Impact on Fraud Investigation and Prosecution
This discussion focuses on the application of fraud detection algorithms, real-time data analysis, and predictive analytics in the context of fraud investigation and prosecution.
Fraud detection algorithms are computational techniques that aim to identify patterns and anomalies in data to detect fraudulent activities.
Real-time data analysis involves the continuous monitoring and analysis of data streams to identify and respond to fraudulent activities as they occur.
Predictive analytics applications leverage historical data and statistical models to predict future fraudulent behavior and assist in proactive fraud prevention measures.
Fraud Detection Algorithms
Fraud detection algorithms play a crucial role in identifying and preventing fraudulent activities by analyzing large datasets and detecting patterns indicative of fraudulent behavior. These algorithms use various techniques, such as anomaly detection, to identify transactions that deviate significantly from the norm and are likely to be fraudulent.
Here are four important aspects of fraud detection algorithms:
- Data preprocessing: Before applying fraud detection algorithms, data preprocessing is essential to clean and transform the data. This step involves removing duplicates, handling missing values, and normalizing the data to ensure accurate analysis.
- Feature engineering: Fraud detection algorithms rely on the creation and selection of relevant features that can capture patterns indicative of fraudulent transactions. Feature engineering helps improve the accuracy of the algorithms by providing meaningful input variables.
- Model selection: Various machine learning algorithms, such as decision trees, logistic regression, and neural networks, can be used for fraud detection. The selection of an appropriate model depends on the characteristics of the dataset and the desired level of accuracy.
- Evaluation and monitoring: Fraud detection algorithms need to be regularly evaluated and monitored to ensure their effectiveness and adaptability. This involves assessing the algorithms’ performance metrics, such as precision, recall, and F1 score, and making necessary adjustments to improve their performance over time.
Real-Time Data Analysis
To ensure prompt and accurate analysis, the timeliness and efficiency of data processing and analysis are crucial in real-time data analysis.
Real-time fraud detection requires the ability to analyze vast amounts of data as it is being generated, allowing for immediate detection and prevention of fraudulent activities.
With the advancement of technology and the availability of big data, organizations can employ sophisticated fraud prevention techniques that leverage real-time data analysis.
By continuously monitoring and analyzing incoming data, organizations can identify patterns and anomalies that may indicate fraudulent behavior.
Real-time data analysis enables organizations to respond quickly to potential fraud incidents, minimizing financial losses and reputational damage.
It also allows for the implementation of proactive measures to prevent future fraud incidents, further enhancing the effectiveness of fraud prevention efforts.
Predictive Analytics Applications
Predictive analytics applications are widely used in various industries to analyze historical data and make accurate predictions about future outcomes.
In the context of fraud detection, these applications play a crucial role in identifying fraudulent transaction patterns and improving the effectiveness of predictive modeling for fraud detection.
By analyzing large volumes of historical data, predictive analytics algorithms can identify patterns and anomalies that indicate potential fraud.
This enables businesses to proactively detect and prevent fraudulent activities before they cause significant financial damage.
Additionally, predictive modeling techniques allow organizations to develop sophisticated fraud detection models that can continuously learn and adapt to new fraud patterns.
Overall, the use of predictive analytics applications in fraud detection helps businesses stay one step ahead of fraudsters and safeguard their financial interests.
Frequently Asked Questions
How Can Big Data Be Used to Detect and Prevent Fraud?
Uncovering patterns and utilizing predictive modeling techniques are key strategies for detecting and preventing fraud. Through the analysis of large volumes of data, big data can provide valuable insights and identify suspicious activities, ultimately enhancing fraud detection and prevention efforts.
What Are Some Common Techniques Used to Analyze Big Data and Uncover Fraudulent Activities?
Common techniques used to analyze big data and uncover fraudulent activities include data mining and anomaly detection. These techniques involve the systematic exploration of large datasets to identify patterns and anomalies that may indicate fraudulent behavior.
How Do Machine Learning Algorithms Contribute to Fraud Detection?
Machine learning algorithms play a significant role in fraud detection by utilizing large volumes of data to identify patterns and anomalies that indicate fraudulent activities. These algorithms can analyze data in real-time, enhancing the efficiency and accuracy of fraud prevention efforts.
What Are Some of the Popular Big Data Analytics Tools and Technologies Used for Fraud Prevention?
Some popular big data analytics tools and technologies used for fraud prevention include machine learning applications and data visualization tools. These tools and technologies aid in the detection and prevention of fraudulent activities.
How Do Real-Time Monitoring and Alert Systems Help in Preventing Fraud?
Real-time monitoring and alert systems, coupled with predictive analytics, contribute to the prevention of fraud. By constantly monitoring transactions and detecting anomalous patterns or behaviors, these systems provide timely alerts to potential fraudulent activities.